Mastering Eigenvalues and Eigenvectors: A Comprehensive Guide
Written on
Chapter 1: Introduction to Eigenvalues and Eigenvectors
Eigenvalues and eigenvectors are fundamental concepts in linear algebra, crucial for various applications in machine learning and data analysis. They provide insights into the characteristics of matrices, which in turn influences how data is processed and interpreted.
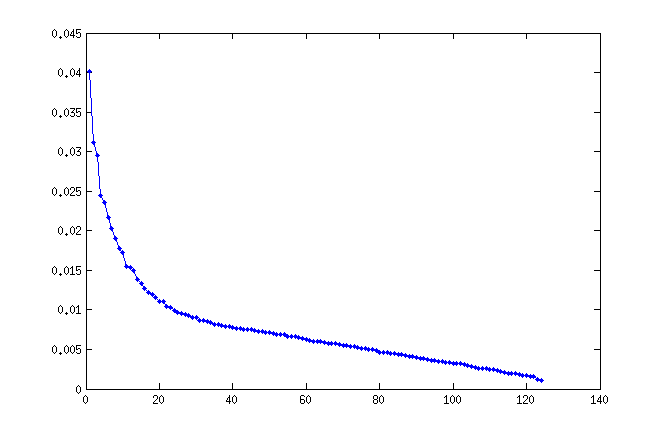
Section 1.1: The Significance of PCA
Principal Component Analysis (PCA) is a technique used for dimensionality reduction and feature extraction. In the realm of machine learning, where data is abundant, efficiently managing features is essential. Feeding a model with every single data point or column can lead to complications and redundancy.
PCA acts as a valuable tool, discerning the most significant independent features from a dataset. To simplify, it provides a representative subset of the entire data, akin to how an elected official represents their constituency. The features selected by PCA indicate their percentage representation of the complete dataset. Let’s delve deeper into PCA’s mechanics, focusing on the calculation of eigenvalues and eigenvectors using a 2x2 matrix.
Section 1.2: Calculating Eigenvalues and Eigenvectors
To illustrate the calculation, we will consider the following matrix A:
A = | 2 6 |
13 9 |
- Step 1: Define ( lambda I ) where ( I ) is the identity matrix:
λI = | λ 0 |
0 λ |
- Step 2: Compute the subtraction of the matrix A and ( λI ):
- Step 3: Determine the determinant of the resulting matrix, yielding:
λ² - 11λ + 60
- Step 4: Set the equation from Step 3 equal to zero and solve for λ, resulting in:
λ = 15, -4
These values are the eigenvalues for the given matrix.
- Step 5: To find the corresponding eigenvector for λ = 15, substitute into the equation from Step 2, yielding:
- Step 6: Multiply the matrix from Step 5 by a vector ( begin{pmatrix} x_1 \ x_2 end{pmatrix} ) and set it to zero:
-13x1 + 6x2 = 0
- Step 7: From this equation, we derive ( x_1 = (6/13)x_2 ). If we assume ( x_2 = 13 ), then ( x_1 = 6 ). Alternatively, if ( x_1 = 0.46 x_2 ) (since ( 6/13 approx 0.46 )), taking ( x_2 = 1 ) gives ( x_1 = 0.46 ). Thus, the eigenvector is ( begin{pmatrix} 6 \ 13 end{pmatrix} ) or ( begin{pmatrix} 0.46 \ 1 end{pmatrix} ).
Repeat this process with λ = -4 to find another eigenvector. This illustrates how to compute eigenvalues and eigenvectors for a 2x2 matrix.
This video, titled "Finding Eigenvalues and Eigenvectors," provides a visual guide to the concepts discussed and illustrates the calculations involved.
PCA and Dimensionality Reduction
PCA is renowned for its role in dimensionality reduction, alongside other techniques like Singular Value Decomposition (SVD). By understanding PCA, we can effectively reduce dimensions while still capturing the essence of the dataset. The proportion of dimensions selected can vary based on specific problem requirements, and tools like the elbow curve can assist in determining which dimensions best represent the dataset.
In conclusion, this overview of PCA and eigenvalue calculations should simplify the coding process and enhance your understanding. Happy learning and coding!
The second video, "Finding Eigenvalues and Eigenvectors: 2 x 2 Matrix Example," further elaborates on the concepts and provides a practical example for better comprehension.