Understanding and Avoiding the Transposed Conditional in Statistics
Written on
Chapter 1: Introduction to Probability Statements
The transposed conditional presents a subtle yet significant misunderstanding within the realm of statistics. Recently, I discussed how to properly grasp statistical significance, and it was noted that I had not addressed the transposed conditional. Thus, this essay aims to fill that gap.
First, I will clarify what probability statements entail. Next, I will delve into how the transposed conditional emerges from incorrect probability statements. Finally, I will propose various approaches to mitigate this issue in practice.
Section 1.1: What is a Probability Statement?
Probability, as a mathematical principle, is partially intuitive but can also be perplexing. We subconsciously engage in probabilistic reasoning on numerous occasions. For instance, when we hastily decide to sprint for a train, we are optimizing our chances of success.
However, we often misjudge the likelihood of rare events. This phenomenon is so misleading that I've dedicated an entire essay to it: How to Perfectly Predict Impossible Events?
A probability statement articulates the mathematical likelihood of an event occurring. Sounds simple, right? Unfortunately, it's not! While absolute probabilities exist in mathematics, most real-world scenarios, particularly statistical ones, require conditional probabilities.
A conditional probability statement articulates the likelihood of an event or observation occurring, given certain underlying information or hypotheses. In probability notation, the chance of observing evidence “E” under the condition that a hypothesis “H” is true is denoted as: P(E|H) — the vertical line signifies “given” or “conditional on”.
For the remainder of this essay, we will concentrate on conditional probability statements due to their practical importance.
Subsection 1.1.1: Illustrative Image
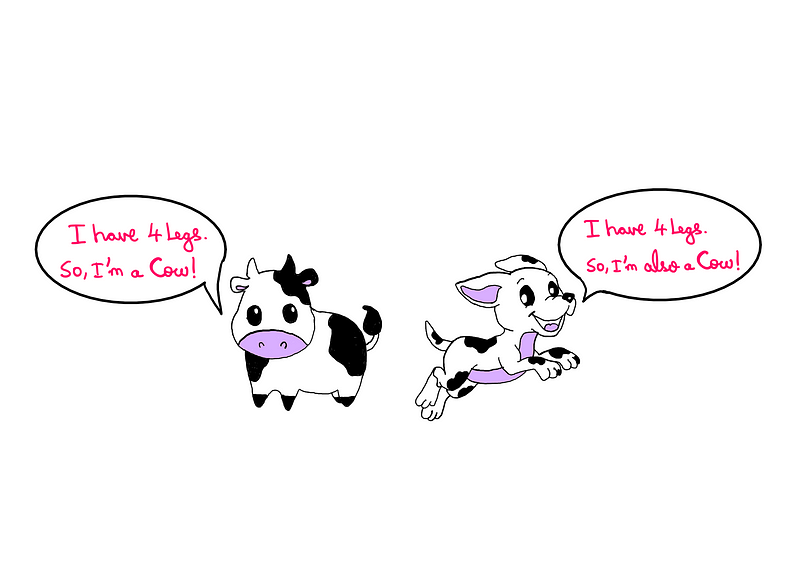
Section 1.2: How Does the Transposed Conditional Arise?
To begin, we need to clarify that in statistics, we frequently encounter statements like:
“The likelihood of observing this result purely by chance is very low.”
Such a statement appears to discuss the absolute probability of an observation. However, it actually conveys a conditional probability under the assumption that the underlying hypothesis holds true. Statistical inferences rarely rely on absolute probabilities; thus, we should presume they are based on conditional probabilities.
The transposed conditional issue occurs when statistical claims inadvertently switch the evidence and hypothesis in practical contexts. Allow me to illustrate this with a simplified example.
Chapter 2: Simple Example of the Transposed Conditional
Imagine we are examining the hypothesis that cows possess four legs, and the animal we are observing is a cow. We might come across these statements:
- The probability that an animal has four legs if it is a cow is one.
- The probability that an animal is a cow if it has four legs is one.
The first statement seems logical based on our hypothesis, but the second appears questionable. It suggests that any four-legged creature must be a cow, which is clearly incorrect.
It's evident that these statements do not convey the same meaning. Yet, in statistical discourse, the second statement is often mistakenly treated as a substitute for the first. This illustrates the transposed conditional.
In more technical terms, let “H” represent the hypothesis that cows have four legs, and “E” denote the evidence of encountering an animal with four legs. These statements can be mathematically expressed as:
- P(E|H) = 1
- P(H|E) = 1
In Bayesian terms, P(E|H) does not equal P(H|E). In this scenario, our focus should be on P(E|H) rather than P(H|E).
Chapter 3: Advanced Example of the Transposed Conditional
Now, let’s consider a more complex scenario. Suppose you’re investigating a crime scene and discover a bloodstain of type “V” that matches a sample from a prime suspect, “Agent X”. Here, “E” signifies the evidence of the bloodstain found at the scene, while “H” denotes the hypothesis that Agent X committed the crime.
You might read these two statements from forensic statistics:
- The probability that the stain came from Agent X if it is of type “V” is 1000 to 1.
- The probability that the stain would be of type “V” if it originated from Agent X is 1000 to 1.
At first glance, both statements seem equally plausible. However, one is incorrect. In mathematical probability terms, they can be expressed as:
- P(H|E) = 1000/1
- P(E|H) = 1000/1
Again, in Bayesian analysis, P(E|H) is not equivalent to P(H|E). We are primarily concerned with P(E|H), making the second statement correct and the first incorrect in this context.
While there may be situations where the roles of these two probabilities are reversed, the key takeaway is that they cannot hold the same probability value. Decisions based on such flawed reasoning can lead to misleading and potentially dangerous outcomes.
Section 3.1: Avoiding the Transposed Conditional
The transposed conditional issue often becomes apparent when examining written statements. However, verbal assertions can easily go unnoticed, leading to misinformation and manipulation.
To steer clear of the transposed conditional, consider the following questions:
- Is the statistical statement indicating a probability concerning the validity of a hypothesis? — If so, be wary.
- Does the statement include explicit conditional qualifiers such as “if” or “given”? — If not, be cautious.
- Is the statement drawing a conclusion without contemplating at least one alternative hypothesis beforehand? — If yes, be cautious.
A well-formulated statistical statement for a general audience might read:
“The evidence strongly supports the hypothesis that the blood stain came from Agent X.”
While this statement lacks explicit qualifiers, it clearly articulates that the evidence bolsters the hypothesis without making claims about the probability of the hypothesis itself.
In summary, whether you are reading or crafting a statistical statement, remain vigilant about the potential transposed conditional and strive to avoid it.
Chapter 4: Further Reading and Support
References and credit: I.W. Evett.
For additional insights, you may find the following articles of interest: The Bell Curve Performance Review System Is Actually Flawed and How to Really Understand the Mathematics of Language?
If you appreciate my work as an author, please consider supporting me on Patreon.
Chapter 5: Practical Video Tutorials
To enhance your understanding of data manipulation in Excel, check out these informative videos:
The first video, 3 Ways to Transpose Excel Data (Rotate data from Vertical to Horizontal or Vice Versa), offers practical tips for efficiently transposing your data.
The second video, Transpose (Flip Around) Data in Excel AND Keep the Formatting ☝️, provides guidance on maintaining formatting while transposing your data.
You can read the original essay here.