DeepMind's AlphaFold 2: Revolutionizing Protein Structure Prediction
Written on
Chapter 1: Understanding AlphaFold 2
DeepMind's AlphaFold 2 has made significant strides in predicting the 3D structures of proteins from their amino acid sequences, achieving levels of accuracy that rival traditional experimental methods.
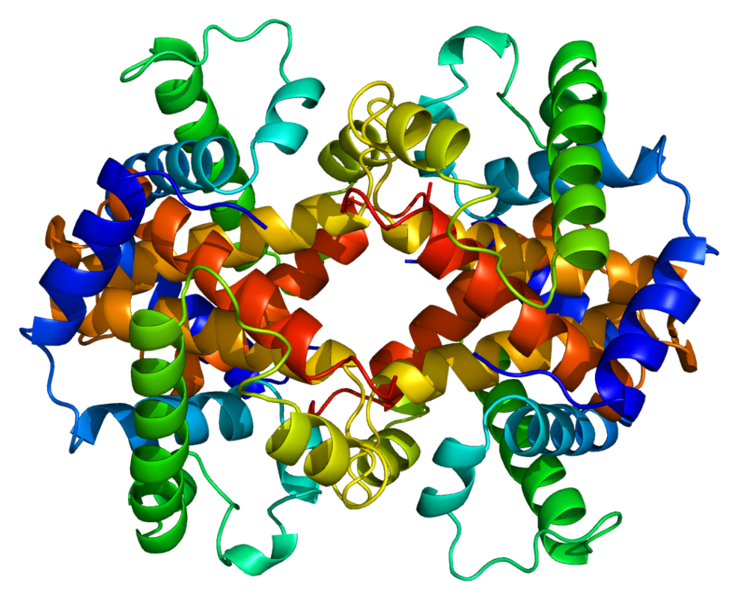
The hemoglobin (HBE1) protein (Wikimedia commons, Emw)
A Challenge Accepted
If you have any interest in the sciences, you may have encountered discussions surrounding DeepMind's remarkable advancements. Their AI, AlphaFold 2, has successfully deciphered how to predict the 3D structures of proteins. Numerous insightful articles exist on this topic, including those from Nature, New Scientist, and the DeepMind blog.
Given my previous explorations into machine learning and AI, I felt compelled to share a brief overview of this latest development. My earlier discussions have covered a range of topics, including the intersection of science and art, historical research, genetic enhancement, mental health, aging research, video game ecology, Hollywood, astrobiology, epidemiology, stock markets, and the job market.
For more in-depth information, consider checking out the articles linked above.
The Story Unfolds
Since 1994, a biennial competition known as the Critical Assessment of Structure Prediction (CASP) has been held to evaluate protein modeling methods. The latest CASP concluded on November 30th, during which DeepMind's team showcased the impressive performance of AlphaFold 2. The predictions made by AlphaFold 2 were found to be comparable to those derived from contemporary experimental techniques.
The efficacy of protein structure predictions is judged using the global distance test (GDT), which measures how closely the predicted amino acid positions align with experimentally determined ones. A GDT score of 90 is deemed the gold standard for experimental results, while AlphaFold 2 achieved scores ranging from 87 to 92.4.
A Protein Interlude
Let’s take a moment to highlight the significance of these advancements.
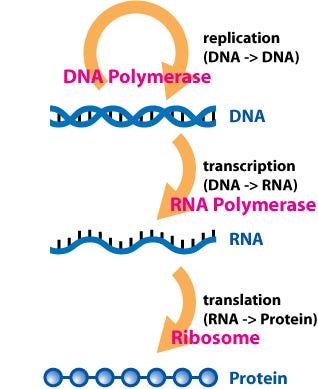
(Wikimedia commons, Dhorspool)
At the core of molecular biology lies the central dogma:
DNA → RNA → Protein.
DNA gets transcribed into RNA, which is then translated into an amino acid sequence. This sequence subsequently folds into a complex 3D structure, culminating in the formation of a protein.
Proteins serve critical roles beyond muscle building; they are the fundamental workers of molecular biology. They regulate metabolism, facilitate DNA replication, signal cellular processes, provide structural support, transport molecules, and respond to various stimuli.
Another vital aspect to note is that a protein's function is intricately linked to its structure. As highlighted in a 2012 review on protein biology:
While Louis Sullivan’s claim that ‘form follows function’ applies to many man-made structures, in protein science, the reverse holds true—function follows form.
Historically, determining protein structure has been performed through experimental methods such as nuclear magnetic resonance, X-ray crystallography, or cryo-electron microscopy. These methods can be prohibitively expensive, taking years and involving significant trial and error.
But, you might ask, since we understand the genetic code—how DNA translates to RNA and then to amino acids—can’t we predict a protein's structure just from its DNA sequence?
The Complexity of Prediction
The term "simply" here is misleading. Predicting how proteins fold is an incredibly intricate challenge. The quest to understand protein structures from amino acid sequences has been ongoing for approximately 50 years. The vast number of potential ways a string of amino acids can fold into a final protein structure complicates matters.
In essence, brute force computation alone cannot effectively explore the myriad folding possibilities.
Enter AlphaFold 2.
According to DeepMind:
"A folded protein can be conceptualized as a 'spatial graph,' where residues represent nodes, and edges connect residues that are in close proximity. For the latest version of AlphaFold, utilized at CASP14, we developed an attention-based neural network system, trained end-to-end, that interprets the structure of this graph. Through iterative processes, the system generates highly accurate predictions of the protein's physical structure within days. We trained this system on publicly available data comprising approximately 170,000 protein structures."
This combination of extensive data and advanced machine learning techniques has led to a groundbreaking achievement.
Looking Ahead
Is this merely hype, or is it a genuine breakthrough? Perhaps it is both.
The real significance—at least in my opinion—is that this development not only represents a landmark achievement but also paves the way for further innovations.
If confirmed, AlphaFold 2's results provide researchers with an invaluable tool to predict protein structures more efficiently and cost-effectively than ever before. This capability is crucial for understanding specific diseases, enhancing drug development, and responding to pandemics.
In the longer term, it could enable the intentional design of custom proteins and even the creation of organic nanobots synthesized by the body.
However, we are not at that point yet.
Looking forward, I envision advancements building on this milestone:
- Predicting Conformation Changes: Many proteins alter their shapes while performing their functions. Understanding potential shape changes could help us explore a protein's full range of capabilities.
- Predicting Protein Interactions: Proteins often form complexes to execute their functions. Can we utilize structural data to forecast which proteins may interact and facilitate reactions?
- Applications in Designer Proteins: Currently, AlphaFold 2 operates on known proteins. Is it feasible to reverse engineer the folding process to create novel "folds" and assign them custom functionalities?
To conclude, here’s a message from the DeepMind team:
"For those of us engaged in computational and machine learning methods in science, systems like AlphaFold highlight the immense potential of AI as a tool for fundamental discovery. The progress announced today reinforces our confidence that AI will become one of humanity’s most valuable instruments for expanding the boundaries of scientific understanding. We eagerly anticipate the many years of rigorous work and discovery ahead!"
Chapter 2: Practical Applications of AlphaFold 2
In this video, learn about using AlphaFold to predict large protein structures with ChimeraX, showcasing the practical implications of this technology.
This video discusses how Google's AlphaFold AI predicts protein structures with unmatched precision, illustrating its potential impact on scientific research.